Transforming business with Generative AI – Four expert insights
GenAI (Generative AI) has created a wave of excitement, empowering individuals to craft top-tier text, graphics, and videos effortlessly.
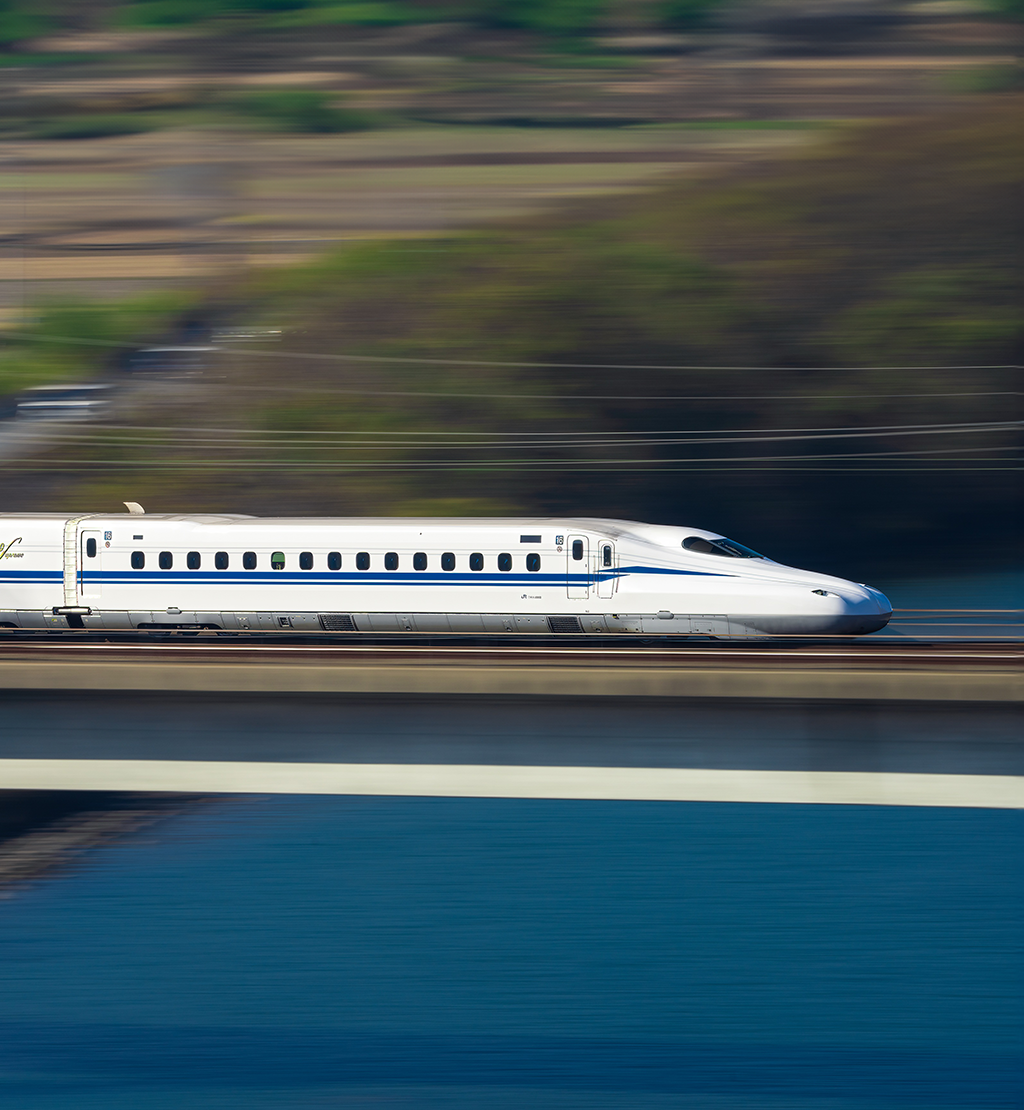
Yet, amidst the buzz, the pressing question remains: How can businesses effectively harness the power of GenAI, and what boundaries does it confront? Jonas Pomoell, AI Lead Consultant at HiQ, shares insightful perspectives on unlocking GenAI’s potential within the business landscape.
At just $5 per month, GenAI may seem like a playful tool designed more for consumers creating shopping lists than generating serious business value. While its primary use may not have been initially envisioned for business applications, HiQ has uncovered some remarkably relevant business cases that demonstrate its unexplored potential in the corporate realm. Here is a glimpse into the valuable lessons learned from these cases.
1: GenAI is indeterministic – Define your quality standards
Whenever Jonas Pomoell, AI Lead Consultant at HiQ in Finland, explains his passion for generative AI, he doesn’t shy away from highlighting its indeterministic nature: “The GenAI model is fundamentally flawed and even chaotic. Instead of providing the correct answer, GenAI gives you a random answer.”
This means that generative models aim to learn the underlying probability distribution of the data they are trained on, allowing them to generate new samples that resemble the training data. When posed with a specific question, GenAI consistently delivers an answer; however, this response isn’t necessarily accurate or optimal – it’s a probabilistic outcome.
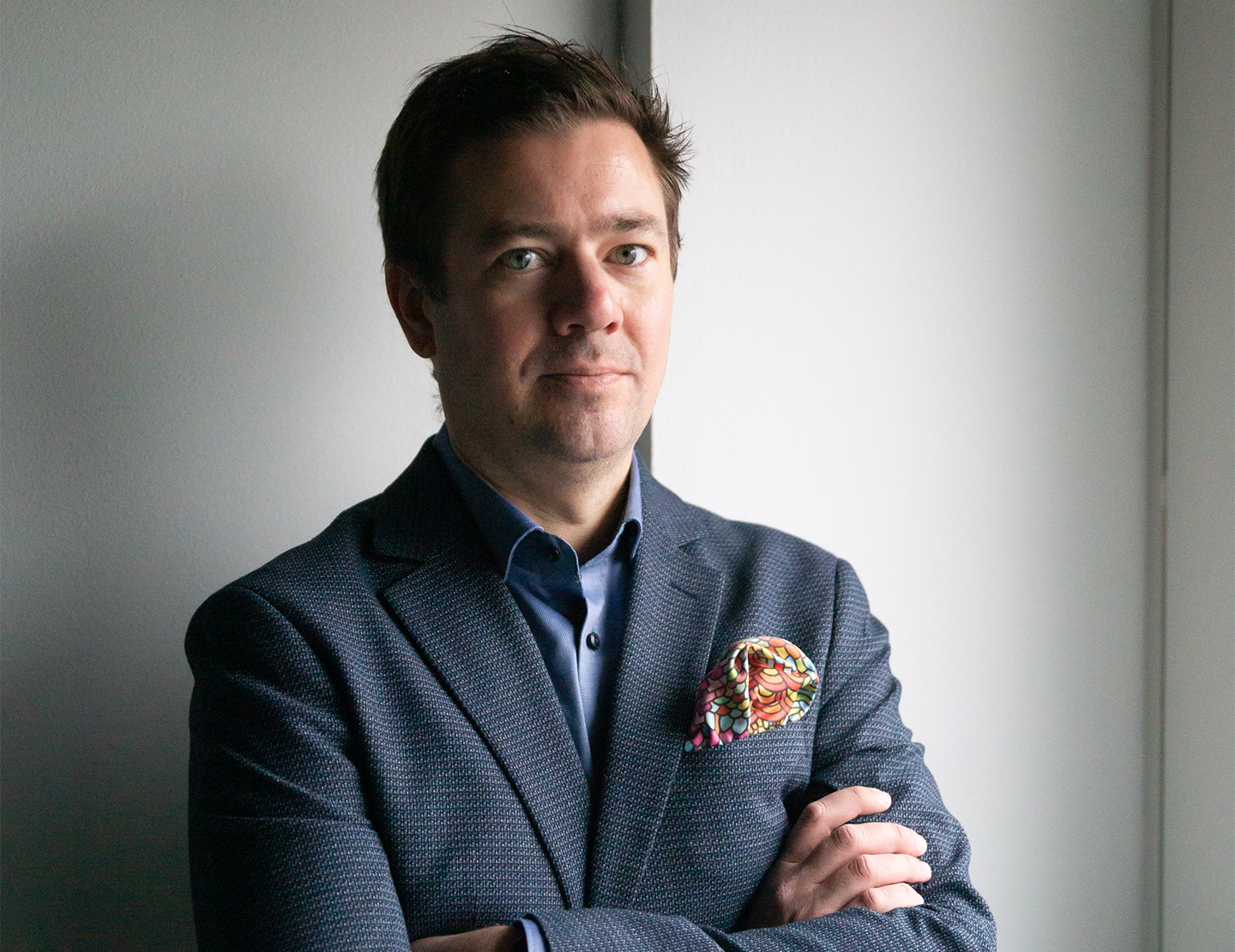
Consequently, effective risk management is crucial when employing GenAI. Companies must precisely define quality standards and the outcomes in the process when answers are incorrect. GenAI can be complemented with human verification, or if specific criteria are not met, answers can be automatically dismissed.
“Risk assessment is crucial – something that we emphasize at HiQ in every scenario. It’s essential to comprehend the potential outcomes if the model fails to perform. For instance, GenAI can act as a handy tool for crafting meta descriptions for online stores. In case the generated text falls short, a human touch can step in for manual refinement. The associated risk is relatively low, and GenAI likely has saved a significant amount of time and effort,” explains Pomoell.
2: Manage your expectations
When it comes to the successful integration of GenAI, effective expectation management is a must. Overly optimistic expectations can undermine the true value of GenAI’s capabilities.
“Consider a scenario where GenAI optimizes 90% of order processing tasks, marking a significant achievement. Sure, the last 10% might need a human touch, but let’s not overlook the massive workload relief GenAI brings to the table. That’s something worth celebrating,” Pomoell says.
Treating this as a learning curve is essential, requiring a readiness for in-depth exploration, discussions, and potential detours in the implementation process.
According to Pomoell, one common pitfall is insufficient planning. Companies eager to adopt GenAI may overlook crucial considerations such as how it aligns with existing IT architecture, its intended use, and the establishment of a clear role for it. Moreover, the implementation of GenAI requires training for employees and potential redesign of services. GenAI, although transformative, does not offer an overnight solution; instead, it reveals emerging development needs.
Time management is another critical aspect. Allocate enough time for processing and maintaining source data, which can take up to half of the development time.
“While creating an AI model might seem straightforward, data processing can often exceed initial estimations. Treating this as a learning curve is essential, requiring a readiness for in-depth exploration, discussions, and potential detours in the implementation process.”
3: Minimize costs by saving data
AI exhibits impressive capabilities in articulating statistics, extracting data, and efficiently handling documents. Pomoell underscores the importance of cost management in every GenAI project.
“When you start planning a GenAI business case, it’s crucial to bear in mind that costs are usage-based. The more generation involved, the higher the costs,” Pomoell emphasizes.
In the realm of GenAI, costs are based on tokens, the smallest units of text data processed by Large Language Models (LLMs). Tokens can take various forms – they might represent characters, words, or even larger text chunks like phrases, depending on the model. Costs can accumulate, with prices like 0.15 cents per 1000 tokens, a substantial amount for industrial players dealing with massive data volumes.
Therefore, strategic planning involves designing the system to gather data concurrently as you progress, eliminating the need for re-generation.
“Throughout the process, it’s advisable to bear in mind that generation is both slow and expensive. Saving the results along with comprehensive metadata is a wise practice. This approach enables you to re-analyze and re-process the results, ultimately saving both time and money. Remember, the more you ‘generate,’ the more it costs,” says Pomoell.
4: First things first – Start with commercial models
Before delving headfirst into a GenAI project, it’s crucial to recognize that thoughtful planning can be a major cost-saving strategy. To begin, Pomoell advises validating your hypothesis with commercial models before committing substantial investments.
“In the experimental phase, go for the cheapest and quickest way to test your ideas; you never know if it’s going to work. Once the project is up and running, creating real value, the costs of GenAI become clear, and that’s when it’s smart to focus on reducing expenses.”
Often, adopting an in-house model featuring a distinctive value proposition proves to be a viable approach. A proprietary model, fine-tuned with unique data tailored to a specific use case, becomes highly resistant to replication. For some companies, possessing a model with Intellectual Property Rights may be business-critical and even create a so called “unfair competitive advantage”.
Businesses creating their own models can establish unmatched competitive advantages, possessing attributes that are exceedingly challenging for competitors to replicate.
“Businesses creating their own models can establish unmatched competitive advantages, possessing attributes that are exceedingly challenging for competitors to replicate. GenAI stands as an excellent choice for pioneers aiming to leap ahead of the competition,” concludes Pomoell.
Key takeaways for your GenAI initiative
- GenAI is indeterministic. Define concrete indicators of the quality of the solution – this is how you know if the project is achieving its objectives. High-risk cases cannot be automated but need human verification.
- Data will make you work. Pay attention to how it fits your architecture as a whole, and remember to train your people. Allocate enough time for processing and maintaining source data, which takes up to half of the development time.
- Small (cost) streams become big rivers fast. Generation is slow and expensive, so save the results and as much metadata as possible – this will allow you to re-analyze and re-process the results, saving time and money.
- Start with a commercial model and invest in your own model only after the project is operational and generating tangible value.
Related content:
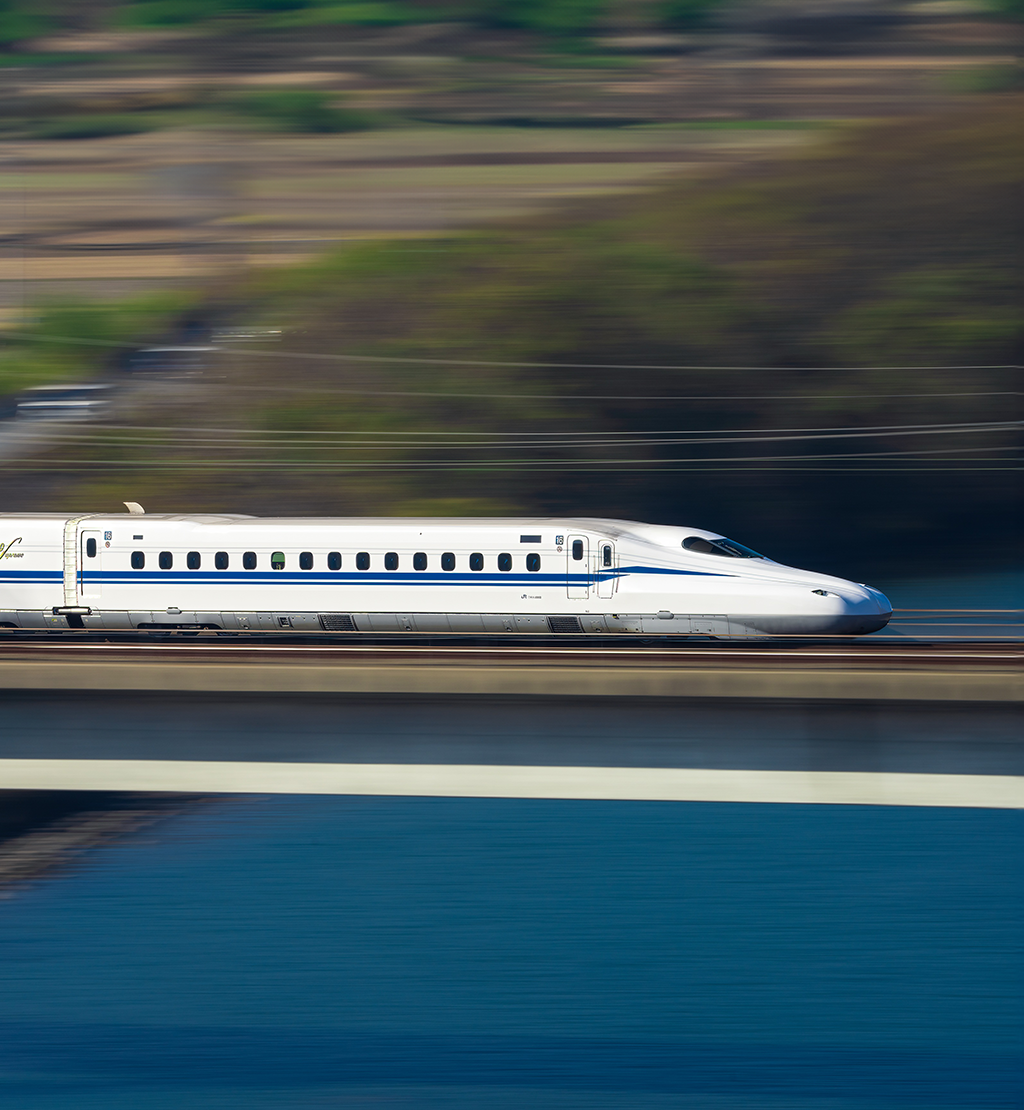
Transforming Business with Generative AI – Four Expert Insights
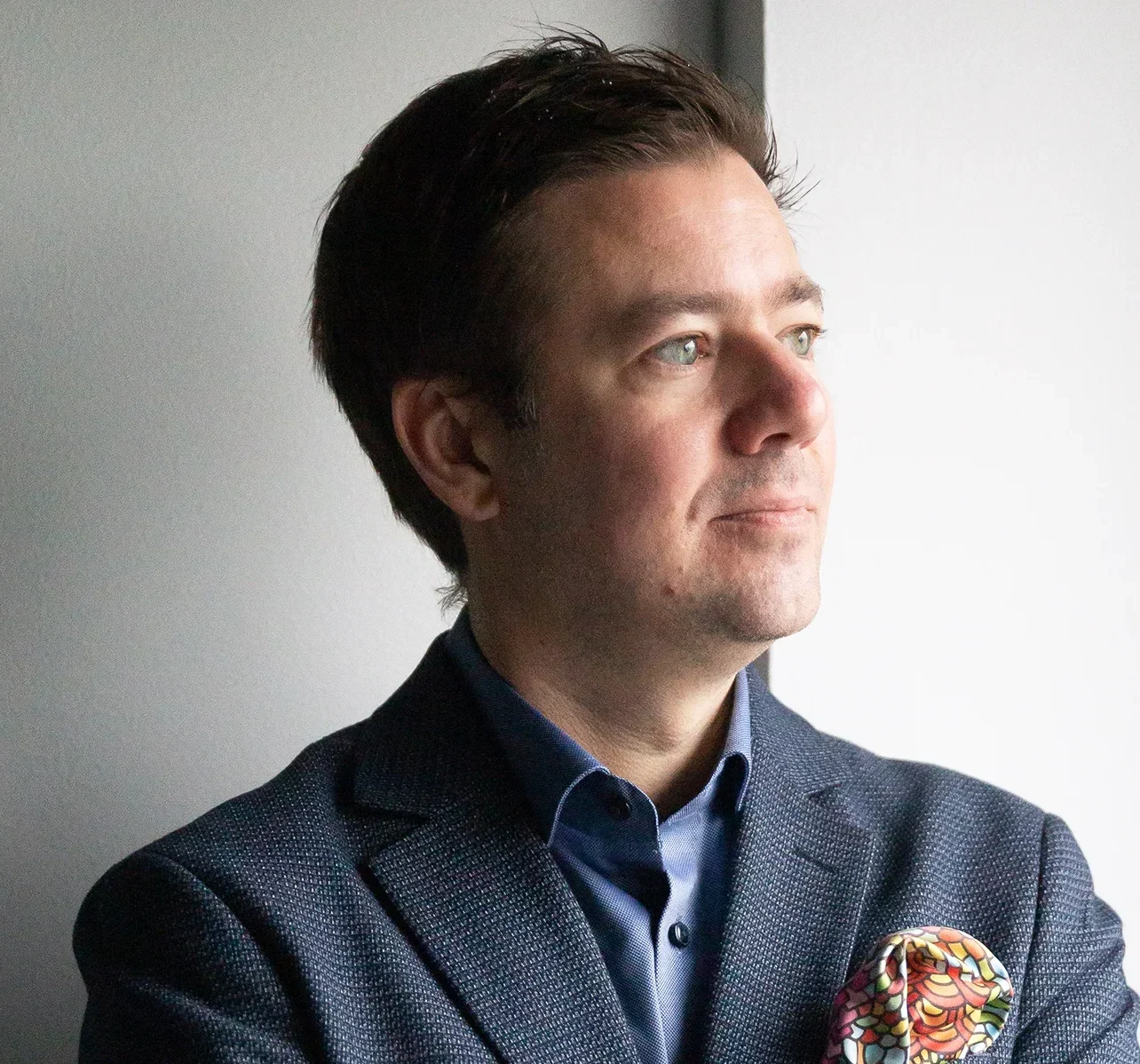
Discovering the potential of coding assistants
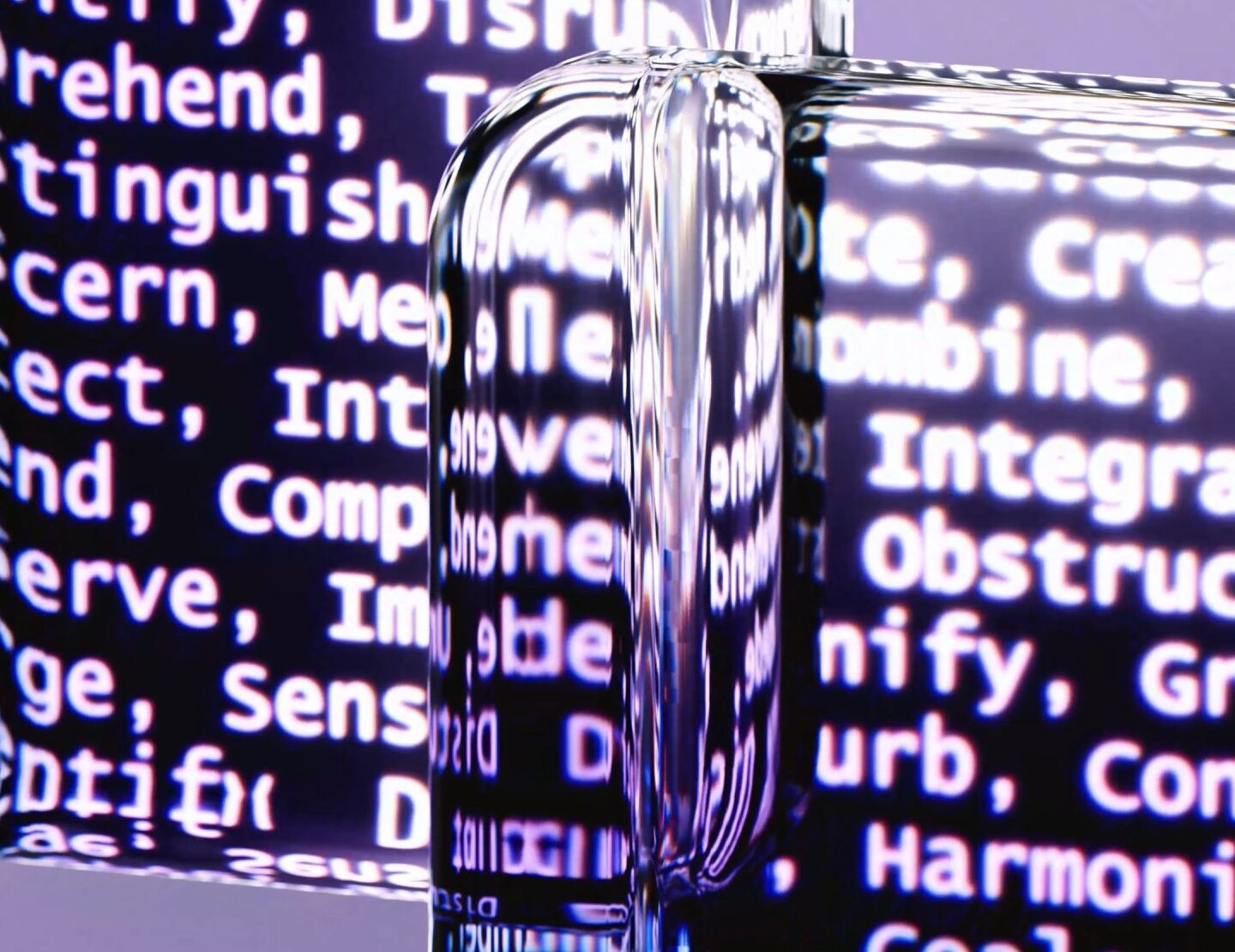
Empower Your Company with a Custom GenAI-Powered Search Engine
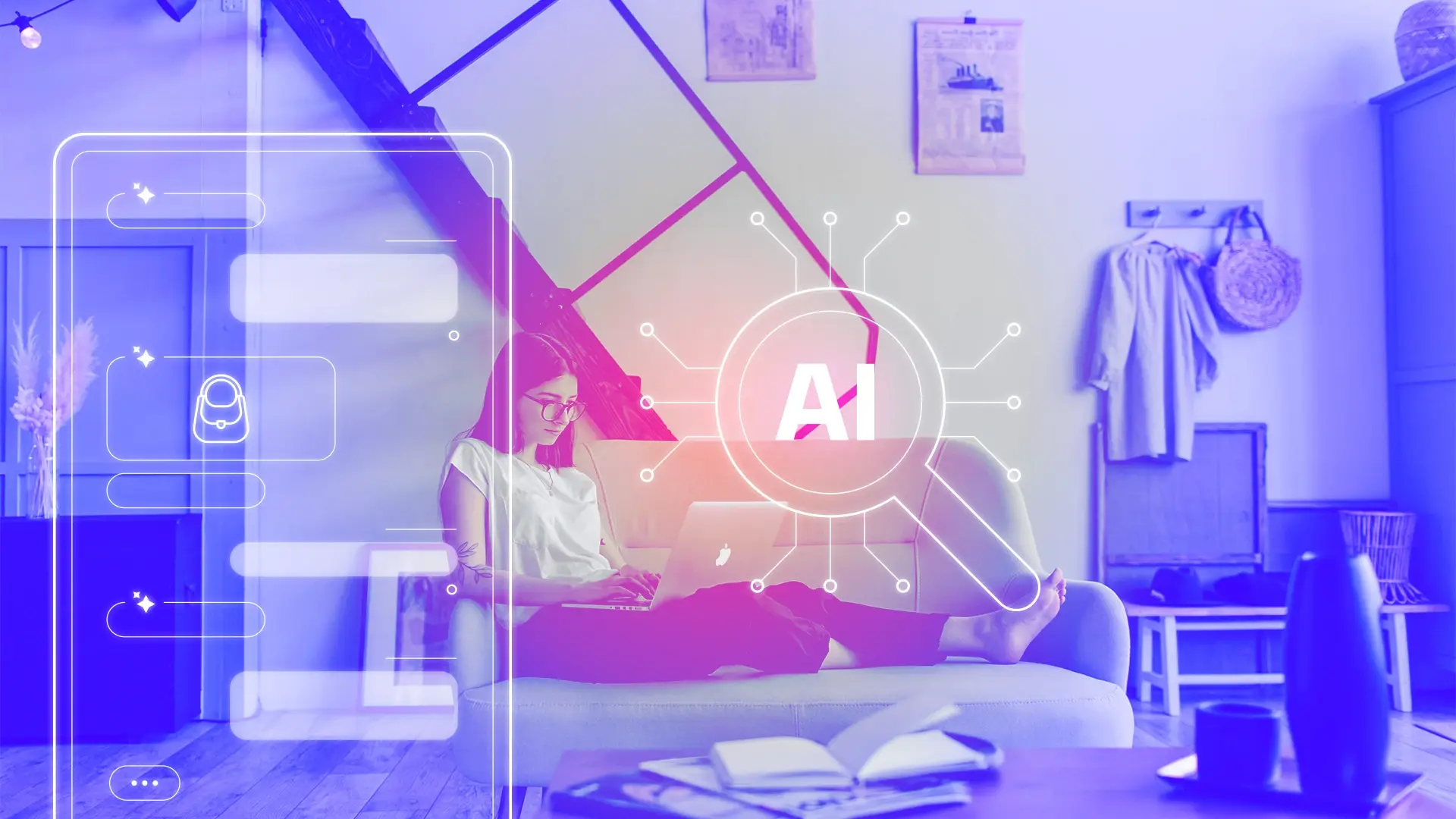
AI knows what you need and orders it for you – purchasing is transforming, here’s how

5 concrete examples of using GenAI search in business
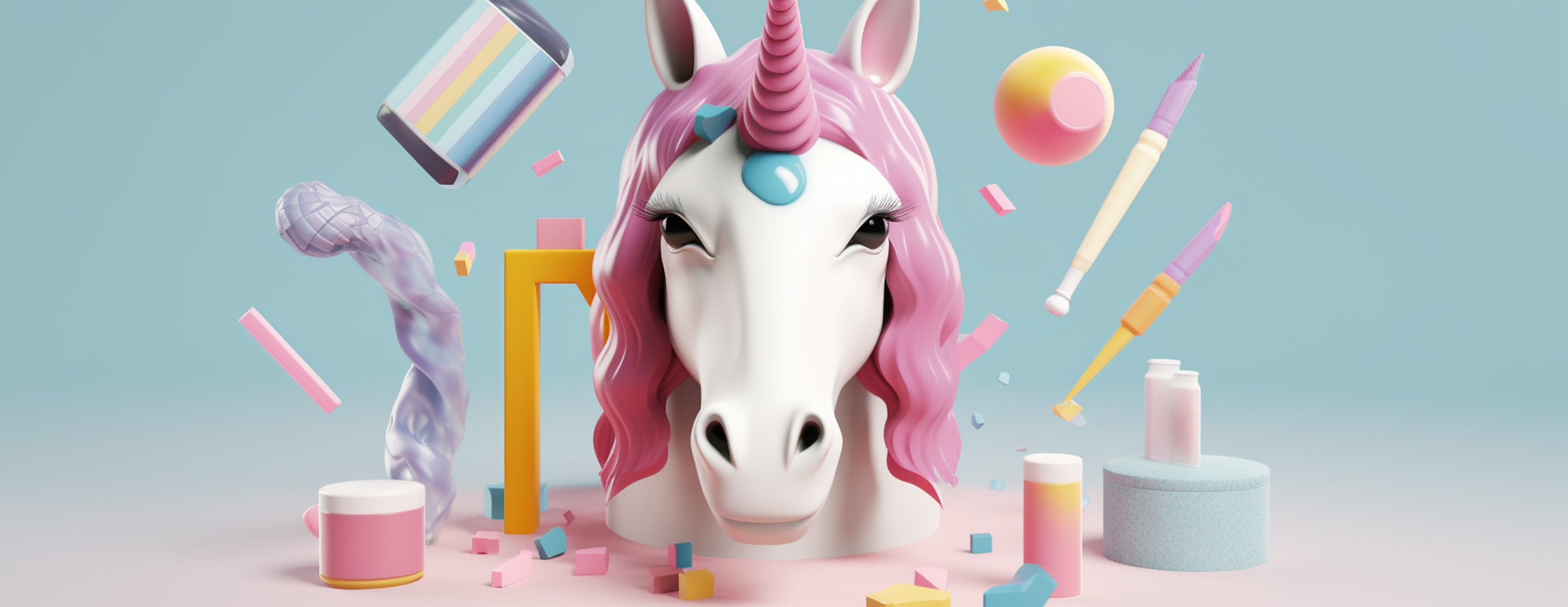